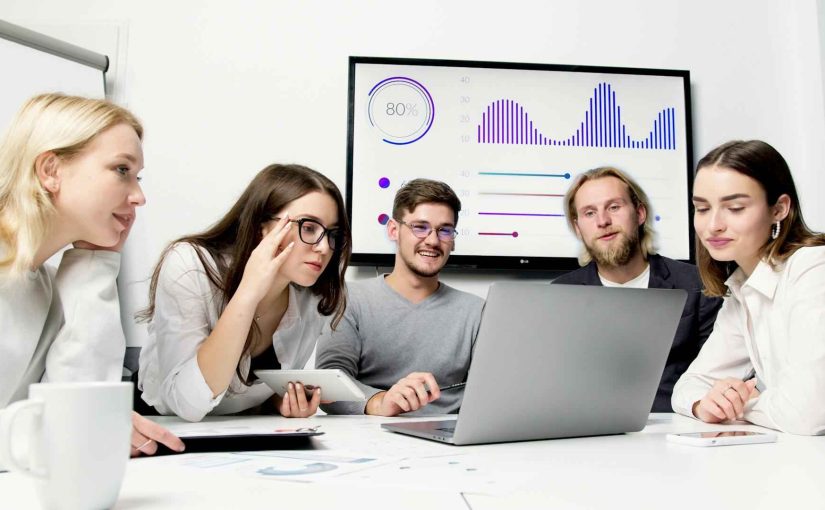
Project risk management is a cornerstone of successful delivery, yet it often remains a challenging and reactive process. The Project Management Office (PMO) plays a vital role in setting standards and guiding projects to success. However, traditional risk management methods often struggle with the increasing complexity of modern projects. AI Project Risk Management offers a more proactive and data-driven alternative, helping teams move from reactive fixes to strategic foresight.
This article explores how Artificial Intelligence (AI) is transforming project risk management within the PMO, making it more proactive, accurate, and efficient.
- The Foundation: PMO and Traditional Risk Management
- How AI Predicts and Mitigates Risks in Project Management
- AI-Powered Capabilities in Detail
- AI Project Risk Management in Action: Case Studies
- The AI Marketplace: Tools and Platforms
- Strategic Roadmap for AI Adoption
- Essential Steps For Successful AI Implementation
- The Future of AI and PMO Risk Culture
- Conclusion
The Foundation: PMO and Traditional Risk Management
The PMO is an organizational unit responsible for defining and maintaining project management standards across an enterprise. Its functions vary from supporting project teams to strategic planning and governance. Key objectives include standardizing processes, improving success rates, aligning projects with business goals, and optimizing resources. In multi-project environments, the PMO maintains an overview, ensures data accuracy, and supports strategic decisions. This central position makes the PMO uniquely suited to oversee risk management.
Traditional project risk management includes identifying potential risks, analyzing them either qualitatively or quantitatively, and planning responses to reduce threats or capitalize on opportunities through mitigation, avoidance, transference, or acceptance. Finally, it involves continuously monitoring risks, addressing new ones, and evaluating the effectiveness of response actions.
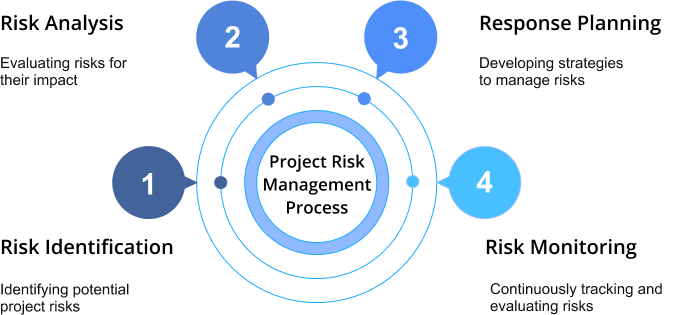
Despite this, traditional methods have significant limitations:
- Incomplete Data: Lack of historical data for new or unprecedented projects.
- Uncertainty and Complexity: Difficulty predicting future events and managing interconnected risks.
- Behavioral Biases: Cognitive biases can distort risk assessments.
- Lack of Integration: Risk management is often siloed from other processes.
- Difficulty Quantifying Intangible Risks: Challenges in measuring risks like reputational damage.
- Reactive Nature: Risks are often addressed only after they materialize.
- Assumption of Risk Objectivity: Risk can be a social construct shaped by perceptions, challenging standardized approaches.
These shortcomings highlight the need for more advanced approaches, paving the way for AI.
How AI Predicts and Mitigates Risks in Project Management
Artificial Intelligence (AI), particularly Machine Learning (ML) and Natural Language Processing (NLP), is fundamentally changing how PMOs manage risks.
Machine Learning (ML) algorithms learn from data to make predictions without explicit programming. In risk management, ML analyzes historical project data, risk registers, and real-time metrics to identify patterns, predict risks, and suggest mitigation strategies. It can handle both structured and unstructured data.
Natural Language Processing (NLP) enables computers to understand human language. It’s invaluable for extracting insights from unstructured text data like project documents, emails, and meeting minutes, identifying potential risks or concerns.
The combination of ML and NLP allows AI to process vast amounts of data and uncover insights hidden in human language. This addresses the struggle of traditional methods with unstructured data.
AI directly addresses traditional risk management hurdles:
- It analyzes vast, diverse datasets to overcome incomplete data issues.
- Algorithmic analysis provides more objective assessments, mitigating behavioral biases.
- Predictive analytics enable proactive risk identification and early warnings.
- Advanced models analyze and model complex risk interdependencies.
- Integration of data from various systems provides a holistic risk view.
- NLP and correlation analysis can provide indicators for intangible risks.
- Automation of tasks improves adherence to risk management processes.
AI also democratizes sophisticated risk analysis techniques and shifts risk management from periodic exercises to a continuous, dynamic process.
AI-Powered Capabilities in Detail
AI equips PMOs with powerful capabilities across the risk management lifecycle.
1. Automated and Insightful Risk Identification
ML algorithms analyze historical project data to identify patterns and correlations that precede risk events. For example, ML can flag early indicators of budget overruns or schedule delays by recognizing trends in past projects. This moves beyond generic checklists to data-driven identification.
NLP enhances this by extracting insights from unstructured data like contracts, reports, and emails. AI-powered NLP can understand context and discern sentiment, identifying emerging themes of concern that might indicate risks. If team communications show consistent negativity about a supplier, NLP can flag this as a potential risk.
AI can even identify “unknown unknowns” by detecting anomalies and significant deviations from normal project behavior.
2. Predictive and Prescriptive Risk Analysis
Predictive analytics, powered by ML, forecast the probability of risks and their potential impact on project objectives like schedule and cost. This allows PMOs to anticipate future states and take preemptive action.
Specific ML techniques used include:
- Bayesian Networks: Model dependencies between risk factors.
- Decision Tree Algorithms: Model decision paths and outcomes related to risks.
- Neural Networks: Uncover complex relationships to predict risk impacts.
AI also bridges qualitative and quantitative analysis. NLP can analyze textual risk descriptions for sentiment. For quantitative analysis, AI makes sophisticated techniques like Monte Carlo simulations more accessible.
3. Intelligent Risk Prioritization and Response
AI enables sophisticated risk scoring based on factors beyond just probability and impact, such as risk velocity and interdependencies. AI can analyze historical data to provide data-driven prioritization.
For risk response, AI acts as a decision support system. ML models can analyze the effectiveness of past mitigation strategies and recommend optimal responses. AI can also automate monitoring of risk triggers and mitigation plans, even triggering predefined responses when conditions are met. This creates a dynamic and adaptive response system.
4. Dynamic Scenario Simulation for Robust Decision Support
AI enables complex “what-if” scenario simulations to explore the consequences of different risk events and response strategies. AI-driven simulations can model the compounding effects of multiple interacting risks. For example, a PMO could simulate the impact of a supplier delay combined with resource unavailability.
These simulations help PMOs:
- Assess overall risk exposure.
- Identify the most critical risks.
- Test the effectiveness of mitigation and contingency plans.
- Determine appropriate reserves.
- Make resilient decisions under uncertainty.
AI-powered simulations act as “organizational rehearsals,” building resilience and improving strategic decision-making.
AI Project Risk Management in Action: Case Studies
Across diverse industries, organizations are achieving tangible benefits by leveraging AI in risk management.
- Deloitte (Consulting): Deployed AI-based risk assessment models to monitor project metrics and compliance across global engagements. This led to a 25% reduction in project failure rates, improved compliance, and enhanced risk visibility.
- Innominds (Construction): Developed AI-driven visual monitoring solutions integrating with BIM and on-site robotics for real-time data capture and safety monitoring. This resulted in a 20% reduction in safety incidents within six months.
- Microsoft (Software Development): Utilizes AI-assisted project management tools and Copilot to analyze project data, identify bottlenecks, and summarize meetings. Outcomes include a 25% increase in project completion rates and a 30% reduction in time on administrative tasks. Copilot also offers AI-generated risk assessments and suggests mitigation plans.
- Siemens (Manufacturing): Implemented an AI-driven risk management system that analyzes historical data to identify patterns and provide real-time insights with recommended mitigation strategies. This has reportedly helped Siemens achieve a 40% reduction in project risks.
- Finance Sector: While not always specific to PMOs, the finance sector broadly uses AI for risk mitigation, such as fraud detection, credit risk assessment, and market risk analysis. Microsoft’s Copilot saved significant time on internal audit reports.
- Pharmaceuticals: Leverage AI to manage risks in drug discovery and clinical trials, optimizing trial design, predicting patient enrollment, and identifying dropout risks. AI also supports drug repurposing and supply chain risk management.
- Critical Infrastructure: AI improves operational resilience through predictive maintenance in energy grids and transportation networks and predicts cybersecurity risks.
These examples demonstrate AI’s ability to deliver enhanced accuracy, increased efficiency, and proactive interventions, leading to measurable improvements in project outcomes.
The AI Marketplace: Tools and Platforms
The marketplace for AI-powered risk management tools is rapidly expanding, offering everything from fully integrated features in established project management (PM) platforms to more focused applications tailored to specific risk analysis needs.
These tools are transforming how teams identify, assess, and respond to project risks – bringing automation, predictive insights, and data-driven decision-making into the core of project planning.
1. Leading AI-Enhanced Project Management Suites
Below are some of the leading solutions that showcase how AI is being embedded into everyday project workflows:
- Microsoft Project (with Copilot) – Predicts task durations and resource needs, offers AI-generated risk assessments and suggested mitigation plans.
- Asana – Uses AI for “Smart Summaries,” “Smart Fields,” and “Smart Status Updates” that can highlight project risks.
- Oracle Primavera – Integrates AI for data-driven insights, with AI agents analyzing dynamic inputs and generating “what-if” scenarios.
- Planview (with Copilot) – Provides proactive insights, surfaces hidden risks in real time, and supports scenario planning.
- Wrike – Offers “AI Project Risk Prediction” using machine learning to forecast potential delays.
- ClickUp (ClickUp Brain) – Embeds AI for features like the AI Knowledge Manager and AI Project Manager, with customizable dashboards to monitor risk factors.
- Nifty – Provides AI-powered project templates and an assistant that streamlines risk-related planning and monitoring.
- Epicflow – Focuses on multi-project resource management, using AI to identify resource-related risks and predict due dates under uncertainty.
- Smartsheet – The AI Agent offers predictive project insights, identifying bottlenecks and risks with real-time anomaly detection.
The trend is clear: “risk-aware” AI is becoming a standard feature across leading platforms, helping teams at all levels access predictive capabilities without needing data science expertise. This democratization of AI is reshaping how organizations approach risk from the ground up.
2. Specialized AI Risk Management Solutions
While many project management suites now include basic AI features, some platforms are designed specifically to tackle complex and high-stakes risk scenarios. These specialized tools go beyond task prediction and resource allocation—focusing on advanced simulations, document analysis, and real-time compliance monitoring.
RiskWatch : A cloud-based platform using AI and machine learning for real-time risk analysis, automated compliance monitoring, and predictive analytics.
Deltek Acumen (Risk and 360): Offers robust tools for schedule and cost risk analysis, leveraging Monte Carlo simulations and integrating AI to support project forecasting.
NLP Risk Document Summarizer : Uses natural language processing and machine learning to automatically extract and summarize risks from contracts, financial statements, and other unstructured documents.
RiskyProject (Intaver Institute) : Provides both qualitative and quantitative risk analysis, including Monte Carlo simulations, with growing capabilities for AI-assisted insights.
These specialized tools offer deeper analytical capabilities but require careful consideration of integration with existing systems.
3. Emerging Tools for Qualitative Analysis and AI-Driven Lessons Learned
AI is also enhancing qualitative risk analysis and leveraging insights from past projects.
- AI for Qualitative Risk Analysis: Tools like Looppanel and Atlas.ti: use AI to transcribe discussions, tag themes, and perform sentiment analysis on qualitative risk data.
- AI for Lessons Learned Analysis: Platforms like Lessonflow and Raidlog AI use AI to analyze past project data and generate recommendations for new projects.
These emerging applications unlock valuable insights from qualitative assessments and historical project narratives.
Strategic Roadmap for AI Adoption
Successfully embedding AI into project risk management requires a clear, phased strategy. This roadmap guides PMOs through assessing readiness, piloting solutions, scaling adoption, and ensuring continuous governance to maximize AI’s impact while managing associated risks.
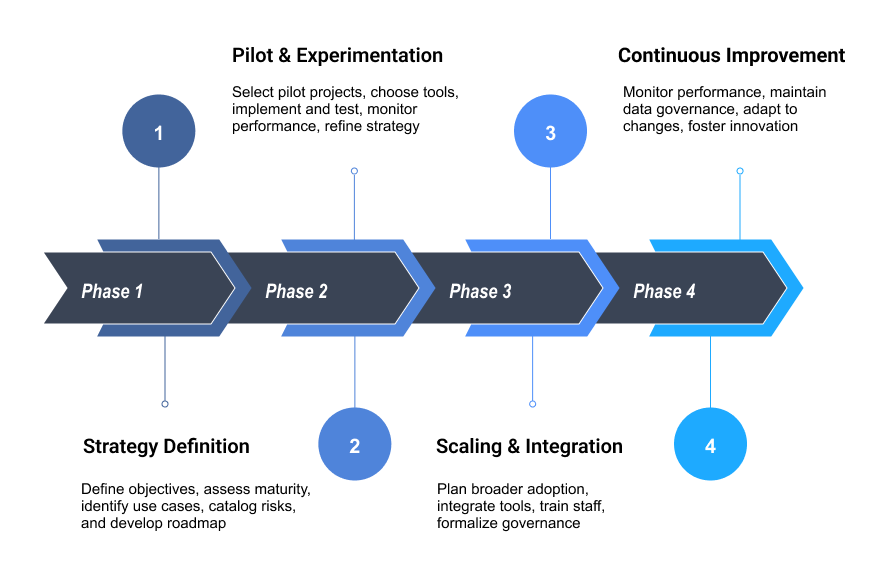
Phase 1: Assessment & Strategy Definition
This initial phase focuses on understanding your current capabilities and setting a clear direction. PMOs define AI goals, assess data and technology readiness, identify valuable use cases, and outline potential AI-specific risks to build a strategic foundation.
- Define clear objectives for using AI in risk management.
- Assess the PMO’s current maturity, data quality, and technology.
- Identify high-impact use cases where AI can deliver value.
- Recognize and catalog AI-specific risks (bias, data quality, security).
- Develop a high-level strategic roadmap for adoption.
Phase 2: Pilot & Experimentation
Here, organizations test selected AI tools in controlled environments to validate impact. Pilot projects provide real-world feedback, helping refine use cases, tool choices, and internal processes before scaling.
- Select pilot projects for initial testing.
- Choose appropriate AI tools based on the use case.
- Implement and test the AI tool in a controlled environment.
- Monitor and evaluate pilot performance against predefined metrics.
- Refine processes and strategy based on pilot learnings.
Phase 3: Scaling & Integration
After proving value in pilot programs, the focus shifts to broadening adoption. This phase includes system integration, organization-wide rollout, staff enablement, and the establishment of robust governance structures.
- Develop a plan for broader AI adoption.
- Integrate AI tools with existing enterprise systems.
- Implement comprehensive training for staff.
- Formalize AI governance structures (data, ethics, model validation).
Phase 4: Continuous Improvement & Governance
Long-term success depends on ongoing optimization and risk oversight. Organizations continuously monitor AI performance, stay aligned with regulatory changes, and promote a culture of innovation and learning.
- Continuously monitor AI model performance and KPIs.
- Maintain robust data governance practices.
- Adapt to evolving AI technologies and regulations.
- Foster a culture of continuous learning and innovation.
This framework, combining strategic direction and iterative experimentation, helps PMOs navigate the complexities of AI adoption.
Essential Steps For Successful AI Implementation
Adopting AI in project risk management isn’t just about selecting the right tools – it’s about laying the operational groundwork for long-term success. To realize the full value of AI, organizations must align technology with strategy, ensure systems work in harmony, maintain data integrity, and lead their teams through change with purpose. Below are the essential components that support a sustainable and effective AI adoption journey:
- Tool Selection: Choose tools aligning with objectives, offering needed functionality, compatible with existing systems, easy to use, scalable, and supported by reputable vendors.
- System Integration: Ensure seamless data flow between AI tools and existing systems.
- Robust Data Governance: Implement processes for data quality, security, privacy, provenance, and ethical sourcing.
- Effective Change Management: Communicate the vision, engage stakeholders, provide training, build trust, and adapt roles and culture.
- Measuring Success – ROI and KPIs: Measuring the success of AI in risk management involves both quantitative and qualitative metrics. Calculating ROI includes direct cost savings and, importantly, avoided costs from successfully mitigated risks.
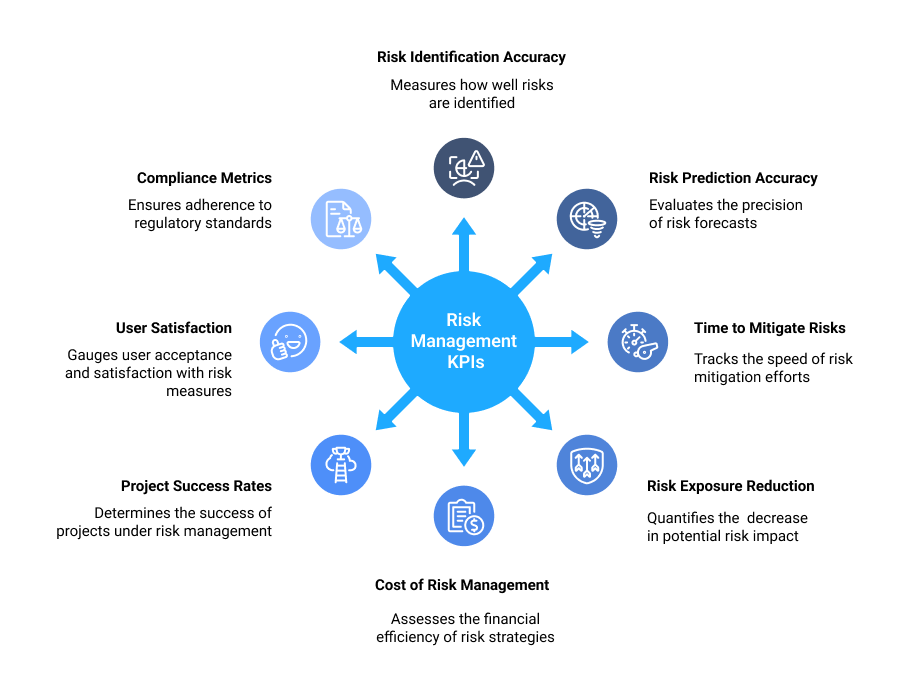
The Future of AI and PMO Risk Culture
The future of AI in project risk management promises increasingly sophisticated capabilities that will fundamentally transform the risk culture within organizations. Generative AI (GenAI) is set to revolutionize risk reporting by automating the creation of natural language summaries and expanding scenario analysis through the generation of a broader range of plausible outcomes. However, careful validation remains essential to address potential inaccuracies.
As AI becomes deeply embedded, the PMO’s risk culture will evolve from a reactive stance to one that is predictive and preemptive. Data-driven decision-making will become the norm, fostering continuous learning, adaptation, and enhanced accountability. This shift will enable PMOs to focus more on strategic risk management rather than just mitigation.
Success metrics for projects will also evolve, placing greater emphasis on risk resilience and adaptive capacity. Meanwhile, cutting-edge research is developing robust AI risk management frameworks and standardized benchmarks to assess AI system risks effectively. Industry analysts predict that AI will soon take over many traditional project management tasks and predict issues with high accuracy, though they warn that poor data quality and inadequate risk controls could lead to project failure.
Ultimately, the future demands that PMOs harness AI’s potential effectively while simultaneously managing the new risks introduced by AI systems themselves.
Conclusion
Integrating AI into PMO risk management marks a pivotal change in addressing project uncertainties. By improving accuracy, efficiency, and proactive insights, AI helps overcome the limitations of traditional risk approaches. Real-world cases show clear benefits across industries.
Success requires more than tools – it demands strategy, data governance, change management, and ethical oversight. Developex partners with organizations to deliver custom software and AI integration solutions that align with business needs and workflows.
Interested in leveraging AI technology for your software projects? Reach out to Developex to discuss how we can help tailor AI solutions for your unique challenges.